Research Articles
DeepONet
DeepONet: Learning nonlinear operators for identifying differential equations based on the universal approximation theorem of operators
- By Lu Lu, Pengzhan Jin and George Em Karniadakis
Generalizing universal function approximators: At the heart of many challenges in scientific research lie complex equations for which no analytical solutions exist. A new neural network model called DeepONet can learn to approximate nonlinear functions as well as operators.
– NATURE by Irina Higgins at DeepMind
Latest Neural Nets Solve World’s Hardest Equations Faster Than Ever Before: Two new approaches allow deep neural networks to solve entire families of partial differential equations, making it easier to model complicated systems and to do so orders of magnitude faster.
- QuantaMagazine by Ani Ananthaswamy
Physics-Informed Learning
Physics-informed machine learning: Physics-informed machine learning integrates seamlessly data and mathematical physics models, even in partially understood, uncertain and high-dimensional contexts.
- NATURE by George Em Karniadakis, Ioannis G. Kevrekidis, Lu Lu, Paris Perdiakis, Sifan Wang and Liu Yang
Physics-informed neural networks: A deep learning framework for solving forward and inverse problems involving nonlinear partial differential equations
- Journal of Computational Physics by George Em Karniadakis, M. Raissi and Paris Perdiakis
Physics-informed neural networks (PINNs) for fluid mechanics: Here, we review flow physics-informed learning, integrating seamlessly data and mathematical models, and implementing them using physics-informed neural networks (PINNs)
- Shengze Cai, Zhiping Mao, Zhicheng Wang, Minglang Yin, George Em Karniadakis
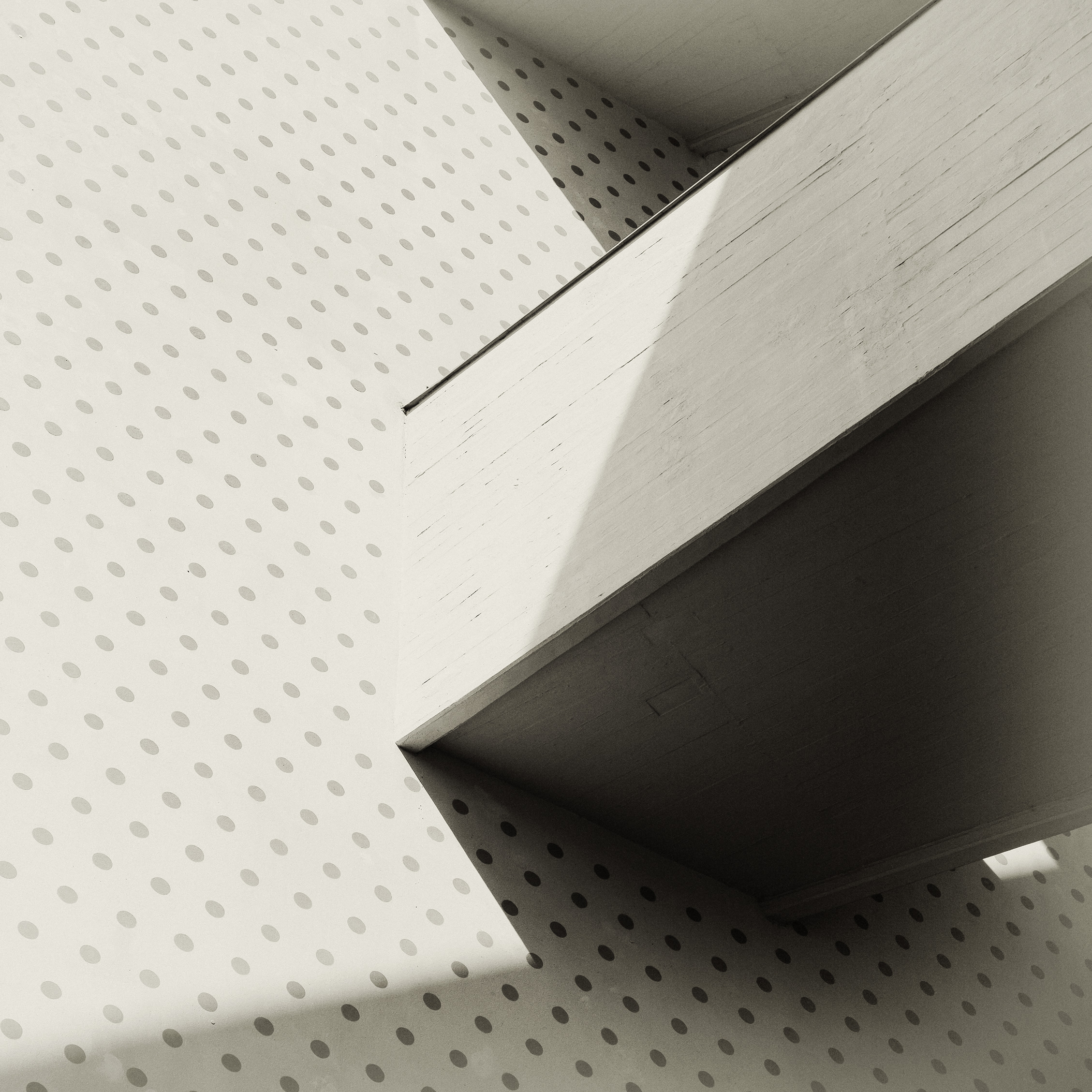